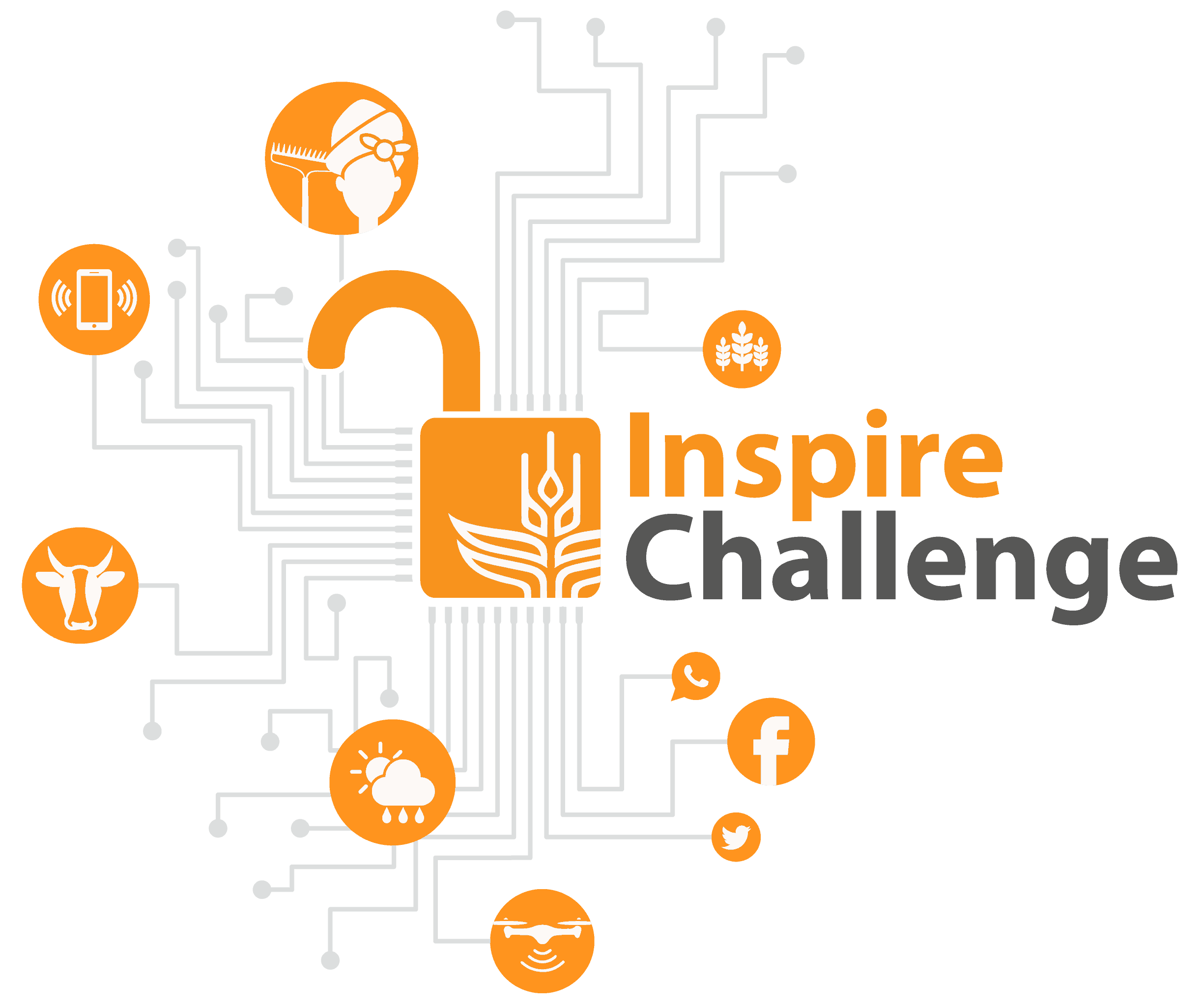
Inspire Challenge 2017 Proposal
Enabling real-time wheat blast management advisories in Bangladesh and Brazil
Though it is the second most important crop globally, sustained wheat production in the tropics and sub-tropics is increasingly challenged by numerous diseases. Wheat blast, caused by Magnaporthe oryzae pathotype Triticum, is a crucial concern.
First discovered in Brazil in 1985, wheat blast since has spread to Bolivia, Paraguay, and Argentina. In 2016, it suddenly appeared outside South America in Bangladesh, and again in 2017 with news reports of further spread to India. Late-season fungicides are only partly reliable. 7 million hectares are at risk of reoccurring infection in South Asia, with losses to smallholders of up to 1.77 MT worth USD 264 million year–1 with light infections of just 10%.
Where extension services can monitor the initial occurrence and climatic conditions, inoculum build-up risk can be assessed and early-season advisories on how to control wheat blast can be deployed.
Protectant fungicides, for example, are most effective when applied before rachis infection occurs. Early leaf infections on Poacea hosts are however microscopic and difficult to distinguish from other diseases.
These challenges emphasize the need for early-season identification to provide reliable advisories.
An idea to solve this
We will develop the Mobile Micro-Disease Detector, a 3-D printable smart-phone clip-on magnifying glass enabling microscopic fungal lesion image collection in the field. The free to download Pic-a-Wheat Field smartphone app, developed by the University de Passo Fundo (UPF) and international partners, will be updated to push microscopic lesion images to the cloud.
Citizen scientists, extension agents and farmers will be mobilized in Bangladesh and Brazil, respectively, to capture tens of thousands of wheat leaf lesion images. Open-source machine-learning image recognition algorithms operating in a cloud computing environment will crunch data at speed and distinguish microscopic wheat blast leaf lesions from tan spot and spot blotch. Resulting data will be presented in real-time as disease distribution maps on Pic-a-Wheat Field, which will be upgraded to provide crop management advisories.
Our hypothesis is that by enabling citizen scientists to collect tens of thousands of microscopic leaf lesion images for rapid identification confirmation by cloud-based machine learning algorithms, farmers and extension services will be empowered to make crucial early-season disease management decisions more rapidly, ultimately reducing risk of large-scale crop losses for smallholder farmers.
We will employ disease disruptive data science to deliver the following innovations:
- Mobile Micro-Disease Detector: A 3D printable smart-phone clip-on accessory (3.5 mm diameter, 100 ´ magnification) based on PNNL will enable microscopic leaf lesion image collection in the field.
- Updates to the Pic-a-Wheat Field smartphone application to catalogue and push time- and GPS-stamped microscopic leaf lesion images to the cloud for big data processing.
- Easy to understand protocols and guidelines for citizen scientists to collect disease images at scale.
- An open-source database of thousands of microscopic wheat disease leaf lesion images.
- Cloud-based machine-learning automated image recognition algorithms to crunch big data at speed and distinguish microscopic wheat blast leaf lesions from tan spot and spot blotch, and to confirm observations with climate-based epidemical information. Using data collected from established weather stations, a proven disease risk model will validate diagnoses.
- Algorithms producing real-time and publically accessible disease incidence maps pushed to Pic-a-Wheat Field, indicating geographical disease distribution with accompanying crop disease management recommendations.
The predicted impact
This innovative approach has major implications for the early detection and diagnosis of other crop and even animal diseases, with potential to transform epidemiological studies across the CGIAR and its partners in the global South.
No previous efforts have been undertaken to link microscopic image collection by citizen scientists with machine-learning enabled disease monitoring systems, nor have these ideas been linked to real-time management advisories.
Piloting is taking place during the coming 2017-18 wheat growing season in Bangladesh (2017-18) and Brazil (2018).
If successful, this disease disruptive data science approach will be expanded to include microscopic lesions on maize and rice, and validated in Bolivia, Paraguay, India, and Pakistan through CIMMYT’s ongoing R4D collaborations.
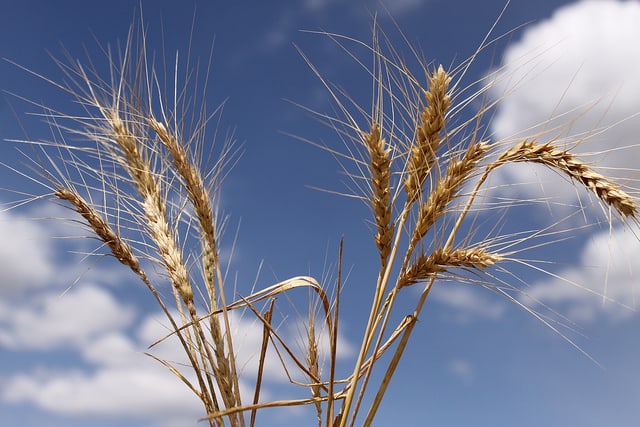
Support this Proposal
The Platform for Big Data in Agriculture is all about the creating connections to build the capacity’s need to inspire real change in the agriculture sector.
The aim of the Inspire Challenge is to generate innovative ideas that will revolutionize the future of agriculture using big data tools. You can be a part of the revolution by supporting these initiatives and help secure food for the future.
Contact one of the team members below to ask how you can help realize this idea.